Writing Questions
With the proliferation of DIY product insights tools, it’s easier than ever to create and send surveys to your users. Writing good study questions that produce valuable data is a bit trickier, though.
When it comes to writing study questions, some people just don’t know where to start. Others make the mistake of believing survey or study questions are simple to write, only to end up with data they can’t use, or worse: data they can use, but which they don’t realize is incomplete or misleading due to errors in question design. The good news is that writing an effective survey or study question is very doable if you follow a few simple best practices. This article covers three basic rules for writing effective study questions. Let’s dive in!
Keep it Simple
The most basic and yet most powerful piece of advice everyone should follow is to keep your questions simple. By following this guideline alone, you can avoid many of the common errors we’ll cover in the rest of this article and series, as well as maximize your response rates and the quality of your data.
Seems easy, right? But it can be more challenging than it sounds. Here’s how to make sure you’ve got it down:
Use easy-to-understand words and phrases.
Regardless of where you’re delivering your studies to users, you’re competing for their time and attention. That means you need to make your questions really easy to understand at-a-glance. Use short, simple words and phrases and aim for a third-grade reading level where possible (think: Charlotte's Web and not War and Peace). This is especially important when trying to boost response rates for studies delivered in-product.
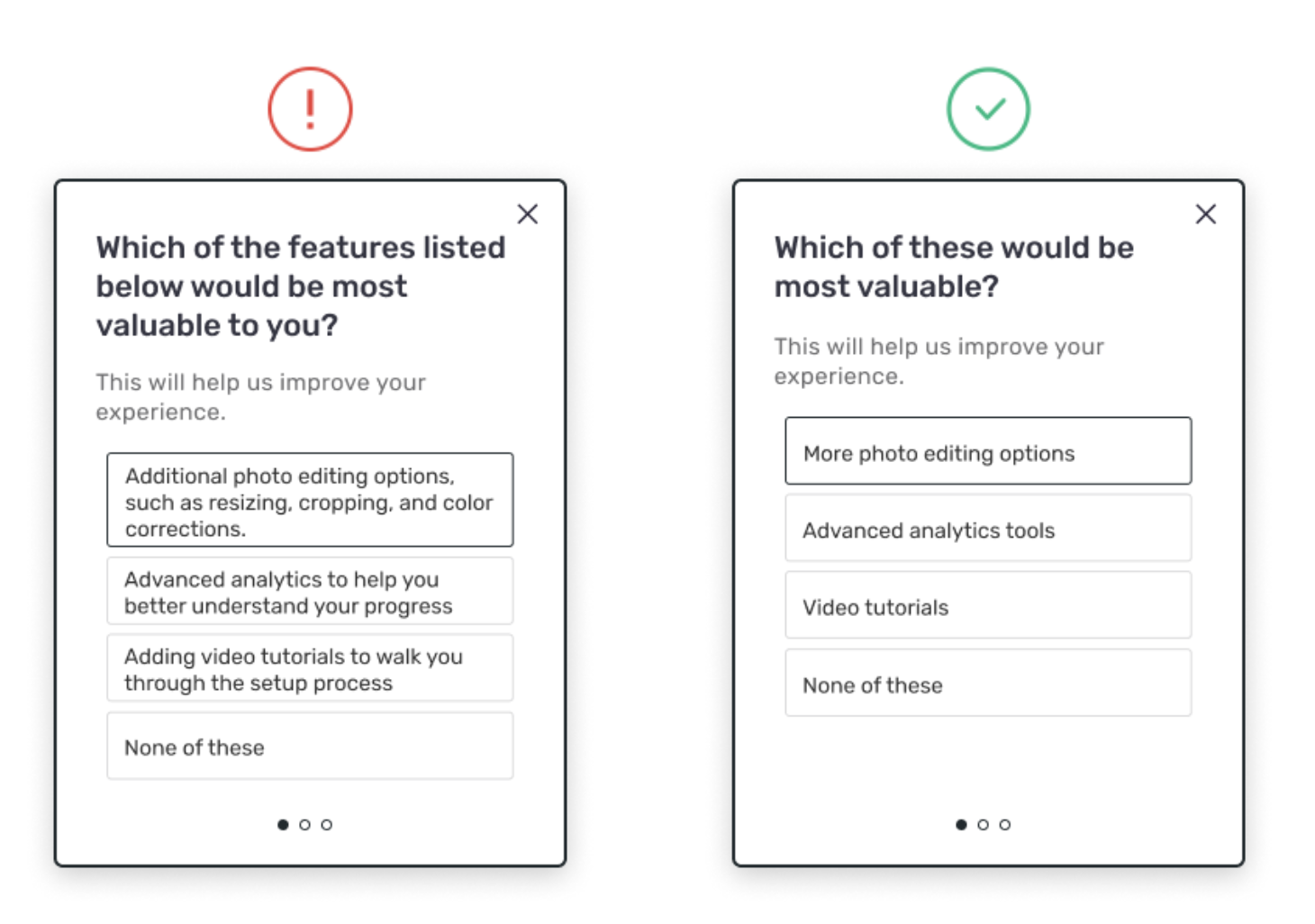
Avoid jargon (e.g., technical language, industry terms, corporate-speak).
It’s very easy to slip into shop talk when drafting study questions if you’re not paying attention. You’re swimming in it all day long and you forget that everyone else isn’t. This includes esoteric technical terms, but also corporate speak -- so avoid words and acronyms like “users,” “onboarding,” “B2B,” “SaaS,” etc.
Keep it ‘human’
Sometimes, in an effort to avoid biasing respondents, we jump through a few too many hoops, with the end result being a question that sounds like it was spat out by a machine. It’s true that you want to avoid bias, but you don’t want to sacrifice users’ understanding and participation in order to do so.
Don't Leave Anything Open to Interpretation
Questions that aren’t completely clear make it possible for different people to answer the same question in different ways, leading to inconsistencies in the data. You may get results that look usable, but those results will not accurately represent what you were hoping to learn. Here’s how to avoid it:
Be as specific as possible to reduce ambiguity.
Start by making your question as specific and concrete as you can. Then, ask yourself how a user might misinterpret it. You’ll often be able to come up with a few possibilities and then you can reword your question to avoid these potential misinterpretations.
For example, maybe you have a two-sided marketplace with buyers and sellers. You decide to gauge loyalty by asking your sellers “How likely are you to recommend our marketplace to friends or family members?” (See the article on Net Promoter Score for more on that topic). You get your results back and you realize you don’t know how to interpret them: are they responding with their likelihood to recommend your marketplace as a place for other sellers to sell their goods, or as a place for buyers to shop? Or are they trying to split the difference, muddling the results even further?
The solution is to make your question more specific. If you care most about sellers attracting buyers to the marketplace, then change your question to: “How likely are you to recommend our marketplace to friends or family members as a place to shop?”
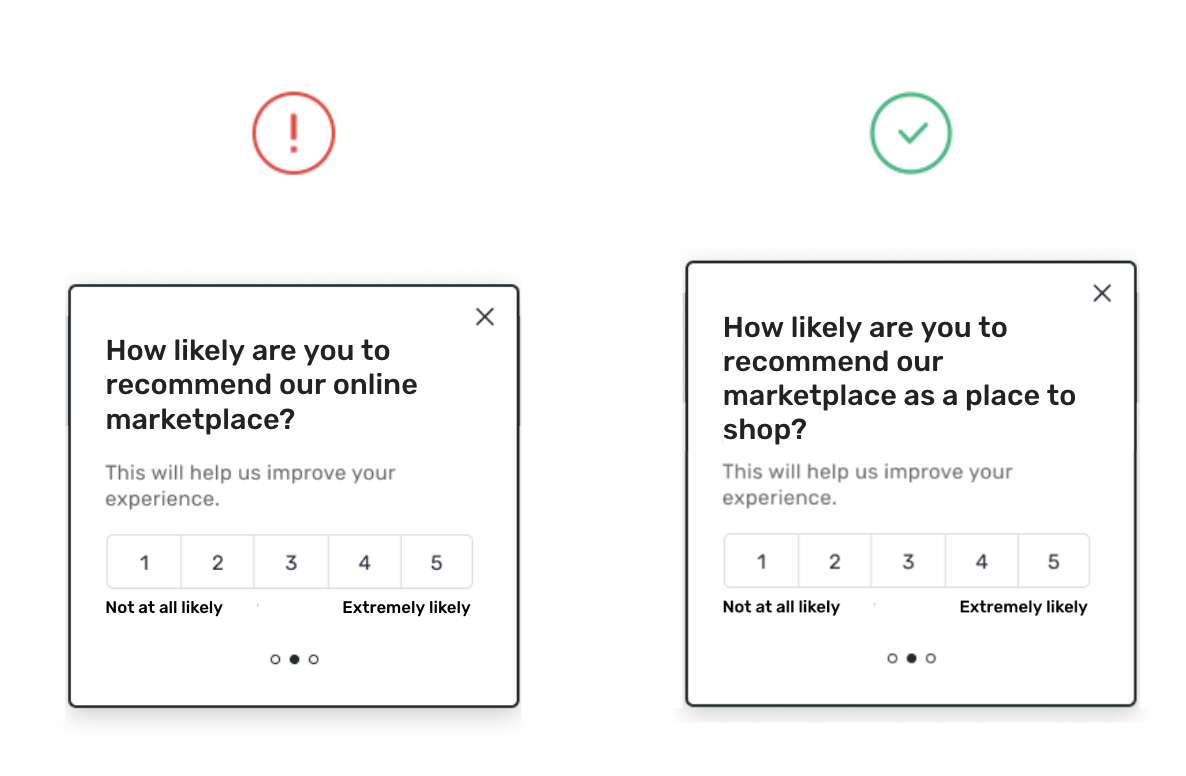
Look out for double-barreled questions.
A double-barreled question tries to cram two questions into one. After writing your questions, check to see if you’ve used the words “and” or “or” in your question text. If you have, there’s a good chance it’s double-barreled.
For example, imagine you just updated your checkout flow and you want to gauge the user experience. You ask, “How fast and easy was it for you to check out?”
You get the results back and they are disappointing. You need to improve, but you’re not sure where to start -- is the problem that checkout isn’t fast or that it’s not easy? Or is it both?
You may argue that you don’t care about the difference -- you don’t need to know if it’s speed or ease that makes the checkout experience bad, you just need to know how you’re doing. Fair enough, but both non-specific and double-barreled questions have negative implications for the quality of your data.
If a user thinks your checkout process was easy but slow, how should she answer the question? If a user would recommend a marketplace to shoppers, but not to other sellers, how should he respond? Different users may take different approaches, making the data that results from your study less than reliable. You may see large fluctuations in results over time or simply obtain results that aren’t quite accurate. The best approach is to make sure your questions are specific and cover only one topic, to begin with.
Avoid Overlapping Answer Choices
It’s possible to leave things open to interpretation in your answer choices as well, such as when answer choices aren’t mutually exclusive. This manifests in different ways, but the most basic example is an overlap in age buckets (e.g., What is your age? 18-35, 35-50 instead of 18-34, 35-50). Users who are 35 will end up spread across both buckets in the first example, but will always fall into the second bucket in your second example. If you happen to have a lot of users who are on the cusp of two answer choices, you may end up with a fundamentally inaccurate understanding of who your users are.
This can also happen inadvertently if your answer choices aren’t specific and participants feel two different choices mean the same thing. That’s why it’s important to stick to all of these rules in your answer choices in addition to the question text.
Avoid Incomplete Answer Choices
Another challenge in the answer choice category arises when your list of options isn’t comprehensive, leaving the user no option to provide an honest answer.
Here’s an example I’ve experienced: “How long is your commute to work? (Less than 15 minutes, 15-30 minutes, 31-60 minutes, 61-120 minutes, N/A I don’t commute)” I can understand why the writers would have thought this is fine, but it just so happened that at the time I took this study my commute was almost 3 hours. In this case, the problem could easily have been resolved by changing the “60-120 minutes” option to “Over 60 minutes.”
This mistake is particularly common with multiple choice questions where participants are given a list of options to choose from. Sometimes it’s just hard to map out all the possible options that exist. The easiest solution to this is to always offer an “Other” or “None of these” option so that there is at least an outlet for participants who can’t find an option that fits them.
Keep Your Eyes on the Prize (Your Goals)
Last, but definitely not least, one of the biggest mistakes I see people make when writing study questions is asking questions that seem interesting, but don’t actually help them make the decisions they are running the study in order to make.
This is an easier trap to fall into than most people realize, but it’s also pretty easy to prevent by adding two steps to your process:
- Clearly define your goals for the study or the 1-2 decisions you plan to make based on the results. Don’t skip this step, even if it seems obvious! If you haven’t laid it out clearly (especially if you’re collaborating with others), it’s easy to throw in all kinds of questions that you won’t actually use, which will lower your response rate without giving you value in return.
- Every time you write a question, ask yourself how the results will help you achieve those goals. What do I mean by this? Look at the question you’ve written and ask yourself: ‘If I learn that X% of users said Y in response to this question, will that help me reach the goal I identified in Step 1?”
You’d be surprised how often the answer is no (that’s why it’s hard to write surveys!). Sometimes the answer is ‘no’ because the question is simply off-target and you need to re-write it. Sometimes it’s ‘no’ because that data point alone won’t have enough context, in which case you may identify an additional question you’ll need to add.
For example, let’s say you want to know whether to prioritize building a Favorites feature in your product and you ask users “Would you like to have a Favorites feature that would let you come back to items you love later?” You get your results back and you see that 85% of users said yes.
At first glance, this seems like a great indicator that you should build a Favorites feature. But the truth is, the results from this question are pretty tenuous, and I would not recommend moving forward on this data alone. This question doesn’t give you any insight into whether those who said ‘Yes’ feel a Favorites feature would solve a genuine problem for them, how valuable it would be to have this feature, or whether they would actually use it. This is the kind of information you’ll need to make a decision.
Here’s an example of a better question: “How often do you find an item that you’d like to save and come back to later? (At least a week, At least once a month, At least once every few months, Once a year or less)”
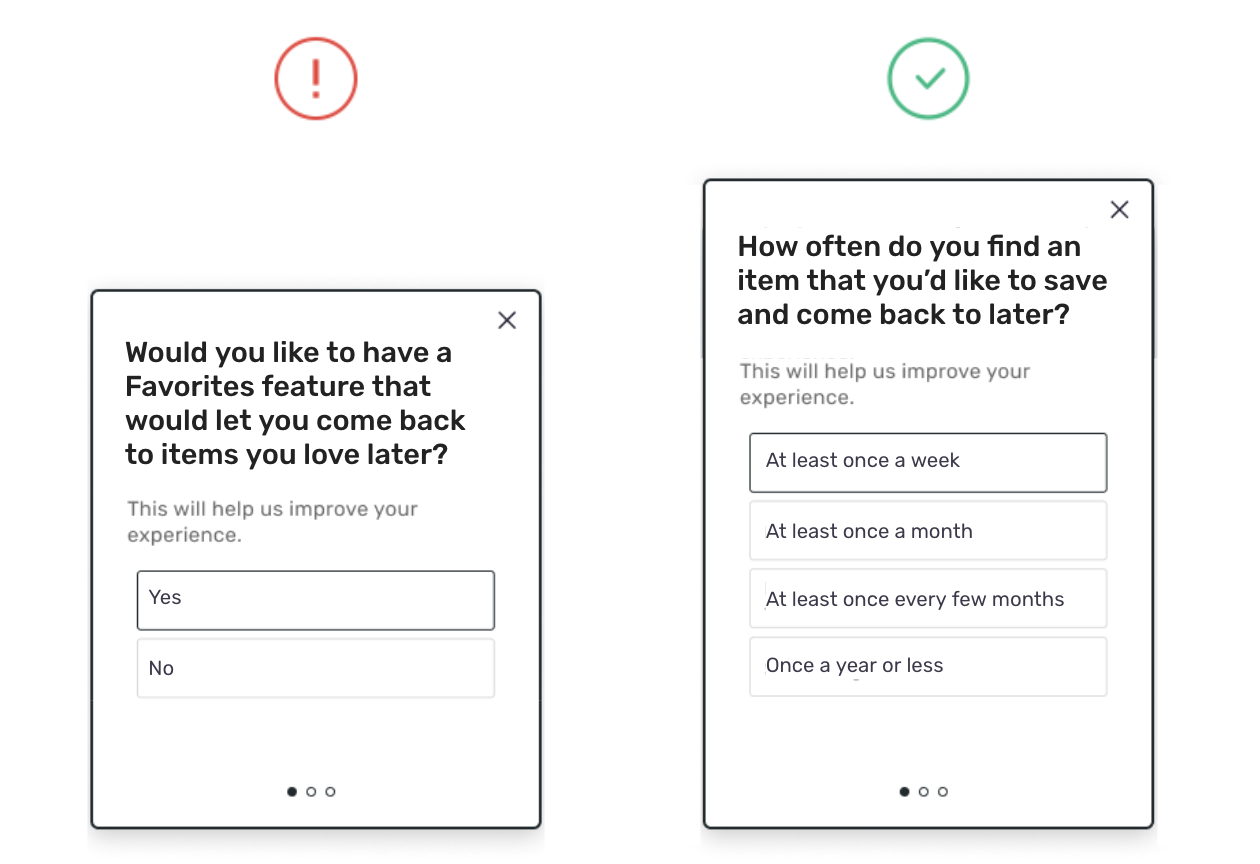
This question asks people about their own experiences, focuses on validating the user problem, and gives us a sense of the value and frequency with which a Favorites feature might be used. If most people say once every few months or less, it’s probably not that valuable, but if most users are saying it happens once a week, now you’ve got something.
Summing it up
There you have it! By following the 3 rules laid out in this article, you’ll be well on your way to creating a high-quality study that gives you data you can act on immediately.
Updated over 2 years ago